Cornell’s RHyME Framework Lets Robots Learn Tasks from a Single How-To Video
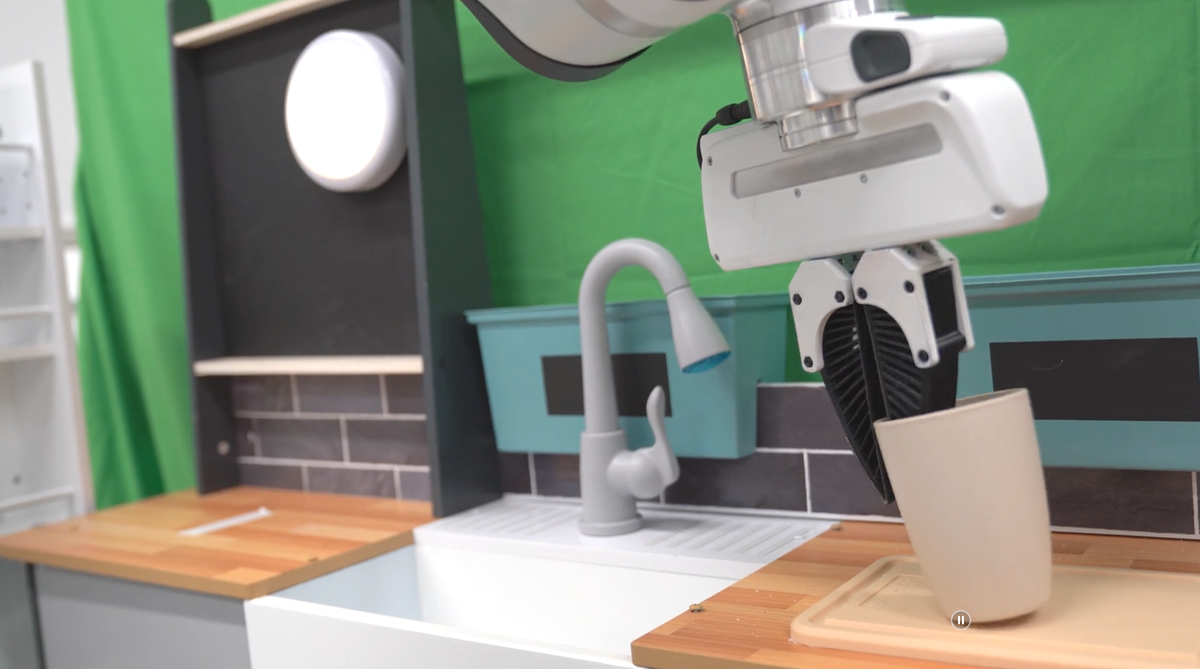
- New AI system boosts robotic learning by using memory and example-based reasoning to overcome motion mismatches.
- Reduces training time to just 30 minutes and increases task success by over 50% in lab settings.
Cornell researchers have developed RHyME (Retrieval for Hybrid Imitation under Mismatched Execution), a breakthrough robotic learning system that enables robots to learn new tasks by watching a single how-to video — and then adapt when things don’t go exactly as planned.
Traditionally, robots need hours of meticulously recorded training data to perform even basic tasks. But RHyME turns that paradigm on its head by allowing robots to watch a single demonstration, then draw on a memory bank of related examples to fill in the gaps — even if their movements or tools don’t match the human exactly.
Trained on just 30 minutes of data, RHyME-equipped robots were able to perform multi-step actions like retrieving a mug or stacking plates with over 50% higher success rates compared to previous methods.
Led by doctoral researcher Kushal Kedia and faculty advisor Sanjiban Choudhury, the system tackles one of the biggest challenges in imitation learning: human-robot mismatch. Unlike older methods that fail if a robot’s motion doesn’t mirror the human demo precisely, RHyME applies a “translation” strategy — interpreting the intent behind an action and finding similar movements it already knows.
The research will be presented at the IEEE International Conference on Robotics and Automation (ICRA) this May in Atlanta, and is supported by Google, OpenAI, the Office of Naval Research, and the National Science Foundation.
🌀 Remix Reality Take:
Forget endless training loops — RHyME is one-shot learning for the real world. By helping robots improvise from inspiration, Cornell’s system makes them more useful, more adaptive, and a lot more human.
Source: Cornell News